THE MARKETER'S GUIDE TO AI
- Home
- THE MARKETER'S GUIDE TO AI
Essential Terms for the Digital Age
Artificial Intelligence is revolutionising marketing faster than any technology since the internet. While 75% of marketers are already using AI tools at work, many struggle to distinguish valuable innovations from industry buzzwords. From automated content creation to predictive analytics, AI is transforming how we attract, engage, and delight customers.
Yet here's the challenge: Only 5% of professionals receive proper AI training, leaving many marketers feeling overwhelmed by technical jargon and uncertain about which AI solutions could truly impact their campaigns. Whether you're considering implementing AI-powered chatbots, exploring predictive lead scoring, or looking to automate your content creation, understanding the language of AI is crucial for staying competitive in 2025 and beyond.
This practical glossary cuts through the complexity, offering clear, marketing-focused definitions of essential AI terms and concepts. We've focused specifically on terms that matter for your marketing strategies, with real-world examples of how each technology can drive ROI and improve campaign performance.
Consider this your practical field guide to the AI marketing revolution – no computer science degree required.
Essential AI Concepts for Marketers
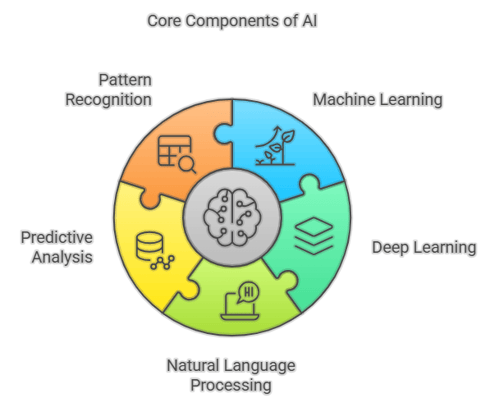
- Artificial Intelligence (AI) in Marketing: Think of AI as your always-on marketing assistant. It's technology that can handle tasks traditionally requiring human marketers - from writing email subject lines to predicting which leads are most likely to convert. While AI excels at analyzing customer data, automating repetitive tasks, and personalising content at scale, it works best when guided by human marketing expertise. For example, AI can draft multiple versions of ad copy, but marketers still need to review and refine the messaging to ensure brand consistency.
- Machine Learning: The engine behind modern marketing automation. Machine learning helps your marketing tools get smarter over time by learning from data. Imagine a system that automatically learns which email send times generate the highest open rates for different customer segments, or which content topics drive the most conversions. In HubSpot, machine learning powers features like predictive lead scoring and content optimisation.
- Deep Learning: The technology that makes advanced marketing personalisation possible. Deep learning helps AI understand complex patterns in customer behaviour, enabling sophisticated applications like:
- Visual content analysis for social media monitoring
- Natural language processing for chatbot conversations
- Customer journey prediction based on behavioral patterns
- Advanced sentiment analysis of customer feedback
- Natural Language Processing (NLP): The technology that helps AI understand and generate human language. For marketers, NLP powers:
- AI-assisted content creation
- Automated email response suggestions
- Social media sentiment analysis
- Chatbot conversations
- Search intent analysis
- Predictive Analytics: The practical application of AI that helps marketers forecast future trends and behaviours. Using historical data, predictive analytics can:
- Identify which leads are most likely to convert
- Predict customer churn risk
- Forecast campaign performance
- Recommend optimal content distribution times
- Project customer lifetime value
- Pattern Recognition: The foundation of AI-powered customer insights. This technology helps identify meaningful trends in your marketing data, such as:
- Common characteristics of your highest-value customers
- Most effective content topics and formats
- Optimal marketing channel mix
- Customer behaviour patterns indicating purchase intent
Understanding these core concepts helps marketers leverage AI tools effectively while maintaining strategic control over their campaigns. Remember: AI is not replacing marketers - it's empowering them to work more efficiently and make data-driven decisions at scale.
AI for Marketing Communications & Content
- Conversational AI for Marketing:
- What it is: Technology that enables natural, personalised conversations with customers at scale
- Marketing Applications:
• 24/7 customer service chatbots integrated with your CRM
• Lead qualification chatbots that pre-screen prospects
• Interactive product recommendation assistants
• Automated appointment scheduling and follow-up - ROI Impact: With 70% of businesses looking to implement AI in customer support, conversational AI can reduce response times by 90% while maintaining personalisation.
- Generative AI for Content Creation:
- What it is: AI systems that create original marketing content based on your brand guidelines and historical data
- Marketing Applications:
• Blog post drafting and ideation
• Social media content creation
• Email subject line generation
• Ad copy variations
• Product descriptions at scale - Best Practice: Use AI for first drafts and research, then apply human expertise for refinement and brand voice alignment.
- Natural Language Processing (NLP) in Marketing:
- What it is: AI technology that understands and analyses human language
- Marketing Applications:
• Customer sentiment analysis from reviews and social media
• Email response classification and routing
• Customer feedback analysis
• Content topic clustering and optimisation - Integration Example: HubSpot's content strategy tool uses NLP to suggest topic clusters and optimize your content strategy.
- Prompt Engineering for Marketing:
- What it is: The art of instructing AI tools to produce marketing-specific outputs
- Practical Applications:
• Creating brand-aligned content briefs
• Generating consistent social media posts
• Maintaining brand voice across AI-generated content
• Customising chatbot responses - Pro Tip: Build a prompt library specific to your brand's tone and style guidelines.
- Semantic Search Optimisation:
- What it is: Advanced SEO that focuses on search intent and context rather than just keywords
- Marketing Benefits:
• Higher quality organic traffic
• Better content relevance
• Improved user experience
• More effective content strategy - Implementation: Modern SEO requires optimising for topics and user intent, not just individual keywords.
- AI Content Strategy Tools:
- What they are: Integrated platforms that use AI to plan, create, and optimize marketing content
- Key Features:
• Content performance prediction
• Topic recommendation
• Automated content briefs
• SEO optimisation suggestions
• Content gap analysis - Platform Integration: These tools often integrate with your existing marketing stack, including HubSpot, to provide seamless content workflow automation.
Remember: The goal of AI in marketing communications isn't to replace human creativity but to amplify it. These tools help marketers focus on strategy and creativity while automating repetitive tasks and providing data-driven insights.
AI Operations for Marketing Teams
- AI Safety & Governance in Marketing:
- What it is: Framework for ensuring your AI marketing tools are reliable, ethical, and brand-safe
- Key Considerations:
• Content moderation for AI-generated materials
• Brand voice consistency
• Customer data protection
• Regulatory compliance (GDPR, CCPA) - Best Practice: Implement clear AI usage policies and review procedures for all AI-generated marketing content
- Algorithmic Bias in Marketing:
- What it is: When AI systems produce unfair or discriminatory marketing outcomes due to biased training data
- Watch Points:
• Customer segmentation fairness
• Ad targeting equity
• Content recommendation balance
• Language inclusion - Prevention Strategy: Regular audit of AI outputs and diverse training data selection
- Human-in-the-Loop Marketing:
- What it is: Marketing automation that combines AI efficiency with human oversight
- Applications:
• Content approval workflows
• Campaign optimisation
• Customer service escalation
• Performance monitoring - ROI Impact: Reduces manual work while maintaining quality control
- Training Data for Marketing AI:
- What it is: Historical marketing data used to train AI systems
- Key Components:
• Past campaign performance
• Customer interaction history
• Content engagement metrics
• Conversion data - Quality Checklist:
• Data accuracy
• Representative sample
• Up-to-date information
• Proper labeling
- Edge AI in Marketing:
- What it is: AI processing that happens directly on devices (phones, tablets, IoT)
- Marketing Benefits:
• Faster personalisation
• Real-time customer interactions
• Reduced data transfer costs
• Better privacy compliance - Example: Real-time personalisation of mobile app experiences
- Marketing AI Implementation Framework:
- Planning Phase:
• Define clear objectives
• Assess data readiness
• Choose appropriate tools
• Set success metrics - Deployment Phase:
• Start with pilot projects
• Monitor performance
• Gather user feedback
• Optimize and scale - Maintenance Phase:
• Regular performance reviews
• Content quality checks
• System updates • Team training
- Planning Phase:
Remember: Successful AI marketing operations require a balance of automation and human oversight. Our AI Excellence Program helps marketing teams implement these concepts while maintaining brand integrity and compliance.
Marketing AI Applications
AI Content Generation: AI technology that creates marketing content including blog posts, social media updates, email copy, and product descriptions. Example: Using AI to generate first drafts of email newsletters while maintaining human oversight for brand voice and accuracy.
Dynamic Content Optimisation: Technology that automatically personalises website content and marketing messages based on user behaviour and preferences. Example: Showing different homepage content to first-time visitors versus returning customers.
Predictive Lead Scoring: AI system that analyses lead data to calculate the likelihood of conversion, helping prioritise sales and marketing efforts. Example: Automatically ranking leads on a 0-100 scale based on behaviour patterns and demographic data.
Smart Segmentation: AI-powered customer grouping based on behaviour patterns, demographics, and engagement data, enabling more targeted marketing campaigns. Example: Automatically creating customer segments based on purchase history and website behaviour.
Real-time Personalisation: Systems that deliver individualised content, offers, and experiences to each user based on their current and historical interactions. Example: Showing product recommendations based on browsing history and similar customer profiles.
Customer Journey Analysis: AI tools that map and analyse how customers interact with your brand across all touchpoints, identifying patterns and optimisation opportunities. Example: Tracking a customer's path from first website visit through to purchase completion.
AI-Powered Analytics: Marketing analytics systems that use AI to automatically discover trends, anomalies, and insights from marketing data. Example: Automatically identifying which combination of marketing channels drives the highest ROI.
Campaign Attribution: AI systems that determine how different marketing touchpoints contribute to conversions, enabling better budget allocation. Example: Understanding the relative impact of email, social media, and paid advertising on sales.
Computer Vision Marketing: AI technology that analyses and optimises visual content like images and videos. Example: Automatically tagging product images for improved searchability or monitoring brand logo usage across social media.
Sentiment Analysis: AI tools that analyse text data to understand customer opinions and emotions about your brand. Example: Monitoring social media posts and customer reviews to gauge reaction to a new product launch.
Marketing Automation Intelligence: AI systems that enhance traditional marketing automation by making decisions about timing, content, and audience selection. Example: Automatically determining the best time to send emails to individual subscribers.
Conversion Rate Optimisation (CRO) AI: AI tools that identify and implement ways to improve website and campaign conversion rates. Example: Automatically testing different page layouts and CTAs to find the highest-performing combinations.
Each of these applications represents a specific way AI can enhance marketing operations. While the underlying technology may be complex, the focus should be on practical business applications and measurable results.
Marketing AI Infrastructure & Platforms
Cloud-Based Marketing AI: Software-as-a-Service (SaaS) platforms that provide AI marketing capabilities through the internet. Example: HubSpot's marketing automation platform with built-in AI features for content optimisation and lead scoring. Benefits include minimal upfront costs, automatic updates, and easy scalability.
On-Premise Marketing AI: Custom AI marketing solutions installed and run on a company's own servers. Typically used by large enterprises that require complete data control and have significant IT resources. Example: A custom-built customer data platform with proprietary AI algorithms.
Hybrid AI Solutions: Marketing platforms that combine cloud-based services with on-premise components. Example: Using cloud-based AI for content generation while keeping customer data analysis on local servers for enhanced security.
Marketing AI Platform: Integrated software that combines multiple AI marketing tools in a single interface. Example: Platforms that combine email automation, website personalisation, and analytics with AI capabilities. Common features include campaign management, content optimisation, and performance tracking.
AI Integration Layer: Software that connects various marketing tools and enables them to share data and AI capabilities. Example: APIs and connectors that allow your CRM to share data with your email marketing platform and analytics tools.
Marketing Data Infrastructure: The underlying systems that collect, store, and process marketing data for AI applications. Example: Customer data platforms that unify information from website visits, email interactions, and purchase history.
AI Development Environment: Tools and platforms used to create and customise AI marketing solutions. Example: No-code AI platforms that allow marketers to create custom prediction models without programming knowledge.
AI Deployment Platform: Systems used to put AI marketing tools into production and make them available to users. Example: Cloud platforms that host chatbots and automatically scale them based on visitor traffic.
AI Governance Platform: Tools for managing and monitoring AI marketing applications to ensure proper use and compliance. Example: Systems that track AI-generated content and maintain audit trails for regulatory compliance.
Marketing AI Stack: The complete collection of AI tools, platforms, and infrastructure used by a marketing team. Example: A combination of HubSpot for core marketing automation, custom AI models for advanced personalisation, and specialised tools for social media analysis.
The key to choosing the right marketing AI infrastructure is matching it to your organisation's needs, resources, and goals. Most marketing teams should start with cloud-based solutions and add complexity only as needed.
Marketing AI Ethics
AI Bias in Marketing: Unfair or discriminatory outcomes in AI marketing systems, often resulting from skewed training data or flawed algorithms. Example: An AI system showing job ads to different demographics based on problematic historical data patterns. Marketers must actively monitor and correct such biases.
Data Privacy Ethics: Ethical principles governing the collection and use of customer data in AI marketing systems. Example: Ensuring transparent disclosure of how AI systems use personal data for personalization and obtaining proper consent for automated decision-making.
Transparency in AI Marketing: The principle of being open and clear about when and how AI is used in marketing communications. Example: Disclosing when chatbots are AI-powered or when content is AI-generated, allowing customers to make informed choices about their interactions.
Marketing Automation Ethics: Guidelines for responsible use of AI in marketing automation to respect customer privacy and preferences. Example: Implementing proper opt-out mechanisms for AI-driven personalisation and maintaining human oversight of automated systems.
Content Generation Ethics: Principles governing the ethical use of AI for creating marketing content. Example: Ensuring AI-generated content is factual, properly attributed, and doesn't perpetuate harmful stereotypes or misinformation.
Customer Profiling Ethics: Guidelines for responsible use of AI in customer segmentation and targeting. Example: Avoiding discriminatory practices in ad targeting and ensuring fair access to products and services across all customer groups.
AI Accountability in Marketing: The principle that organizations must take responsibility for their AI marketing systems' decisions and impacts. Example: Having clear processes for reviewing AI-generated content and addressing potential biases or errors.
Ethical Data Collection: Guidelines for gathering customer data ethically for AI marketing systems. Example: Using only legally obtained data, being transparent about data collection, and respecting privacy preferences.
Human Oversight Principle: The requirement to maintain meaningful human control over AI marketing systems. Example: Having human marketers review and approve AI-generated campaigns before publication and monitoring AI system outputs.
Responsible AI Deployment: Framework for implementing AI marketing tools in ways that respect customer rights and privacy. Example: Testing AI systems for bias before deployment and regularly auditing their performance for unintended consequences.
Remember: Ethical AI marketing isn't just about compliance—it's about building trust with your customers and maintaining your brand's reputation. Always prioritise transparency, fairness, and respect for customer privacy in your AI marketing initiatives.
CONCLUSION
This glossary provides a starting point for understanding the key terms and concepts in the world of AI. As AI continues to evolve, new terms and technologies will emerge. By staying informed and embracing these advancements, you can leverage the power of AI to transform your business and achieve your marketing goals.
Understanding AI jargon is essential for anyone who wants to engage in meaningful conversations about this transformative technology. Whether you're a business leader, a marketer, or simply curious about the future, familiarising yourself with these terms will empower you to navigate the ever-evolving landscape of AI.
Moving Forward with AI Marketing
Marketing is undergoing a fundamental transformation. With 75% of marketers already using AI tools but only 5% receiving proper training, understanding these concepts isn't just helpful—it's essential for staying competitive in 2025 and beyond.
This glossary serves as your practical guide to AI marketing terminology, but it's just the beginning. As AI technology evolves, new capabilities and terms will emerge. We regularly update this resource to reflect the latest developments in AI marketing technology.
The key is not just understanding these terms, but applying them effectively to your marketing strategy. Remember that successful AI implementation isn't about replacing human marketers—it's about empowering them with tools that enhance creativity, improve efficiency, and drive better results.
Ready to put these concepts into practice? Whitehat's AI Excellence Program can help you:
- Assess your AI marketing readiness
- Develop a customised implementation strategy
- Train your team on relevant AI tools
- Ensure ethical and effective AI usage
Contact us to discuss how we can help you navigate the AI marketing landscape and transform your marketing operations for the digital age.
Stay informed about the latest AI marketing developments by joining our monthly AI Marketing Intelligence newsletter or attending our regular AI Marketing Masterclass sessions.
Citations
- Bubeck, S., Chandrasekaran, V., Eldan, R., Gidel, G., & Raynal, P. (2022). A universal law of robustness via isoperimetry. arXiv. https://arxiv.org/abs/2201.11903
- Fogarty, L. (2023). How the context window works in GPT models. Zapier. https://zapier.com/blog/context-window/
- Hardesty, L. (2017, April 14). Explained: Neural networks. MIT News. https://news.mit.edu/2017/explained-neural-networks-deep-learning-0414
- Pasick, A. (2023, March 27). Artificial intelligence glossary: Neural networks and other terms explained. The New York Times. https://www.nytimes.com/article/ai-artificial-intelligence-glossary.html
- Smith, C. S. (2019, November 19). Dealing with Bias in artificial intelligence. The New York Times. https://www.nytimes.com/2019/11/19/technology/artificial-intelligence-bias.html